Sales leaders often come to us to level up their sales messaging, hoping we will download our brains into their sales execution platforms (SEPs) and give them all of the sales messages they could ever need.
While we love writing messaging with sales teams, it’s not enough to have a baller messaging library full of perfect sequences and cadences. This is a launch pad, but, because your prospects—and the market—are constantly changing, your team needs an adaptive way to respond to those changes and iterate over time.
The best way to do that is with a dynamic A/B testing strategy. The trouble is that most sales leaders aren’t sure how to create a framework to guide their teams’ A/B tests.
And when they try to find resources, everything they find is about button placement, user-interface design for apps, and other marketing and product team variables. For a long time, A/B testing has been left to marketing, and it shows in the desert of information out there that’s specific to sales.
To help fill the gap, we’re sharing everything you need to know to create an A/B testing framework with your sales team and the people who contribute to your sales messaging program.
Test your personal best practices and the ones you learn from experts
Your sales career has probably advanced because your personal “playbooks” have worked really well. So it’s only logical that, when you moved into leadership, you (like many other sales leaders out there) have trained your teams to do things the same way.
Here’s the problem: just because it worked for you doesn’t mean it will work for others, or that those strategies were successful for the reasons you think. Without A/B testing assumptions, it’s almost impossible to understand why certain messages (or other strategies) either do or don’t work.
Those tips and tricks are valuable starting points, but they should be tested and either confirmed or adjusted by data. Because sales is such a dynamic art, you might find that a tactic which worked six months ago is no longer effective. Or that a message which resonates with one persona or industry doesn’t work with others.
Here are a few quick examples of tactics that once worked, and are still repeated by many sales teams, but have since passed their prime:
- “Quick question” as a subject line
- Using slides in a discovery call
- Referencing ROI in cold outbound emails
- Asking for time, as a call to action in cold emails
If your team is still doing any of these things, don’t panic. It’s worth “starring” these tactics for one of your first A/B tests. But the truth is that, regardless of what experts (or even outside data) might recommend, what is true for one team may not be true for yours.
“Best” practices almost never apply universally, and the only way to know what works for your team is to experiment as much as possible.
A/B testing experimental design (keyword)
Hopefully by now we’ve convinced you that you should be A/B testing your sales messages. Now it’s time to dive into how to design your experiments.
Step one: Create your hypothesis
A hypothesis is nothing more than a question and a guess at the answer. The “guess” usually has two parts: what you think might be the source of the problem which, if changed, could impact the outcome. The second part of the “guess” is what that impact could look like.
Your A/B test, like any good experiment, is testing whether or not your proposed answer or guess is correct. If it isn’t, then you test another guess until you’re satisfied that you’ve answered your question.
Here are the three parts of your hypothesis, as it should be written out:
- Problem: Articulate the problem either in a brief sentence or question. (This is what’s happening).
- Change: Explain the change (variability) you’re introducing. (I think it might be because of this thing, so I’m going to test that).
- Expectation: Outline your expectations for what you think will happen.
Putting it together, your hypothesis will look something like this:
Problem: Prospects are not replying to our evergreen outbound sequence or cadence at the rates needed to hit our booked meeting targets.
Change: We will test templates one and two, with A-templates asking for a meeting and B-templates asking whether the prospect is interested in learning more.
Expectation: We expect that reply rates in template B will be higher in both email steps.
Here’s how to generate your list of hypotheses
While it might be easier for us to say, “go test these three hypotheses,” your most valuable experiments won’t be canned. They will be based on your own data and context.
To develop your first list of hypotheses (or research questions), we recommend collaborating with your sales messaging creators or sales ops colleagues to see:
- What is your average sales email open rate?
- Reply rate?
- Which sequences or cadences are performing better than that baseline?
- Worse?
- What is your best single email template?
- Worst?
Then, ask “why?” If you can’t explain performance gaps and differences, then it’s time to create a hypothesis (or a set of hypotheses) to answer that fundamental question.
For instance, you might see a big difference between your highest and lowest performing email templates. Look at those templates and see what differences you can isolate. Is one longer? Are the subject lines distinct? Does one have images or hyperlinks?
If you start making some educated guesses, then you’ve identified your first research questions.
Step two: Choose ONE variable
Shortly, we will get into the most common variables sales teams often test. Before we do, though, let’s spend a quick moment reviewing their purpose.
A variable is the change you’re introducing. For example, if your research question has something to do with the length of subject lines, then you’ll want to create two emails to compete against each other.
You will keep both templates exactly the same but change the subject line in your “B” or second template. That altered subject line is your variable. If you change more than that subject line, then you no longer have a reliable variable.
When we work with teams who have A/B tests already running in their SEPs, and we see that they’ve changed more than one variable between two templates or sequences, it makes us feel a little bit like this:
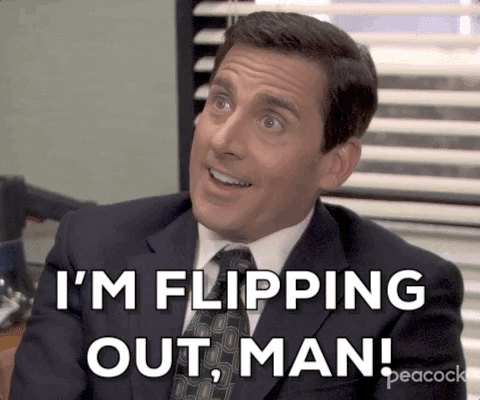
The more variability you introduce into an experiment, the less you can trust the results. This doesn’t mean you can’t have more than two templates competing against each other in a sequence or cadence step (you can).
It does mean that if you’re changing more than one thing across those templates, then you’re doing it wrong. For example, here are two email templates with one difference or change:
Template A:
Hi Erika,
Congratulations on your recent promotion! I saw that you’re now in a VP role, overseeing all of go-to-market.
Does your current marketing automation platform offer the complexity you’re looking for, as prospects move through your funnel?
Would you be interested in talking more about a few ways to eliminate manual steps and offer a frictionless UX?
Thanks,
Dane
Template B:
Hi Erika,
I talk often with other revenue leaders who oversee go-to-market strategies, and I’m curious to hear about how your sales process compares.
Does your current marketing automation platform offer the complexity you’re looking for, as prospects move through your funnel?
Would you be interested in talking more about a few ways to eliminate manual steps and offer a frictionless UX?
Thanks,
Dane
These templates are part of an experiment testing the impact of personalization. Template B could be sent to anyone within her persona, whereas template A was personalized just to Erika.
To monitor this experiment, teams would watch reply rates to see whether the time invested in research and individualization paid off.
If a rep were to change template A’s CTA, in addition to the opening line, then it would throw off the experiment. The new CTA would be just as likely as the personalization to change levels of engagement.
Here are some common variables to choose from:
Subject line
Testing subject lines is often the best way to learn how to increase open rates. A prospect won’t reply or book a meeting frombased on an email they won’t read, so subject lines are critical.
You might test word or character count, including emojis, capitalization, including the prospect’s name or company, asking a question, mentioning a competitor, or countless other ways to evaluate what does and doesn’t inspire prospects to open the email.
Levels of personalization
Testing personalization can be more complicated, as teams often struggle (like in the example above) to change only one element. When reps are given the opportunity to personalize a template, it’s important that they don’t erase the entire template and start from scratch.
By personalizing only the part of the template that’s been highlighted as the variable, you learn whether that personalization was the change that actually made the difference.
Two common spots in a template to test for personalization are the opening and the closing line. Leaving the middle 80% of the email consistent between templates helps to keep the experiment pure.
Other personalization tests can include:
- Using the prospect’s name in a subject line
- Inserting a personalized video versus personalized text
- Inserting a personalized video versus a marketing video or asset
Value propositions
You should never send a sales email without a statement of value. If you’re not telling a prospect what you can do to help them, then they have no reason to care.
But it’s not enough to take a generic value proposition from marketing, drop it in an email, and hit send. Your sales team should be testing value propositions with various personas and industries to see what sticks.
For instance, if you have five key value drivers, you can choose the top two or three which you think will resonate most with your highest-value persona. Create A, B, and even C templates which are otherwise identical but each swap in a different value proposition.
As prospects fitting that persona pass through that sequence or cadence, you will begin to see which one (or ones) best suits that audience.
Marketing assets
As your sales and marketing teams collaborate, both teams benefit from gathering as much data as possible to inform their efforts.
For instance, if your marketing team creates assets (white papers, customer stories, case studies, downloadable assets, one-pagers, landing pages, event registration pages, etc), then it’s important to test how these are resonating with your sales prospects.
Your team might decide to create an A-template that includes a one- or two- sentence summary of a customer story, written in sales language. A B-template might include the same but with a hyperlink to a fuller story OR a question asking if they would like to learn more about what that customer did to achieve the stated results.
Watching the performance of each template can guide marketing to make more of the highest-performing help to create further questions to learn the highest- impact resources for marketing can make to help sales to book more meetings.
CTAs
Calls to action are often the variable with the greatest direct power over reply rates or conversions on specific requests. They can be tricky because messaging teams can be tempted, after one or two experiments, to think they’ve found the magic sauce.
The reality is that personas tend to have different preferences for how directly you approach them with an ask. As they advance in a sales funnel, prospects also tend to have different thresholds and preferences.
As such, teams often benefit from testing CTAs methodically, focusing both on when calls to action fall in a sequence or cadence and who the calls to action go to. After several experiments in both categories, you are likely to be ready for some valuable global testing to determine your team’s best practices for CTAs.
Other variables to consider
Your team might also consider testing out sign-offs, the use of post-scripts, region-specific language, email length, inclusion of images, inclusion of hyperlinks, balance between product-focused language and pain-focused language, tone, and other template-specific variables.
For sequence-wide tests, teams can also clone sequences or cadences and make a single change in the overall sales play. For instance, you might add a call step at the beginning or end of a sequence or cadence. Or, you might test an experimental new tool, like adding a video step or sending swag in the mail.
Step Three: Decide how on the specific way you will measure your results
Evaluating the success of your A/B test will often come down to a few metrics, which are the data points you will watch to see if your guess was correct. The five most common metrics are:
- Opens
- Replies
- Clicks
- Bounce rates
- Conversions on a specific call to action
The part of your hypothesis that outlines your expected outcomes will almost always list one of these metrics as the way to determine whether the change you introduce will have the impact you’re expecting.
Note: We will add the caveat that clicks are rarely the metric of focus, but measuring clicks does make sense if your team is testing whether a certain hyperlinked resource is performing better than another.
Or, if you’re trying to drive traffic to a landing page, then varying hyperlinked CTAs between two templates, with clicks as the determining factor, will help you see what draws people to your page. This is an example of a conversion-based experiment.
How to analyze A/B test results (keyword)
As your experiment progresses, you will watch the target metric in both your A and B email templates, sequences, or cadences to understand if the change you expected is happening. But confidently analyzing results isn’t just observing that your “A” template, sequence, or cadence is performing better or worse than your “B.”
You also need enough sends to be sure the change you’re seeing isn’t due to random chance; this is called statistical significance. It takes a lot of sends to trust your data, and not every team sticks with a test long enough to be confident in the results.
One of the benefits of using a sales execution or marketing automation platform is that it will take care of monitoring this for you, if your experiment is a “race” between two email templates.
While the experiment is running, it will often tell you the experiment is in progress and live report on sends, opens, clicks, and replies going through each experimental template. When the platform is done experimenting, it will give you a report of the test, which often includes:
- Total sends through each template
- Clicks, opens, and replies for each
- How confident you can be in the results
- A winner, citing a specific variable as the reason
However, you can’t always rely on your platform to analyze the results for you. As part of equipping your team with an A/B testing strategy, you also have to teach them how to analyze results on their own.
There may be occasions when your team will need to stop the experiment before your platform is finished running it. Reasons for this might be:
- A time-bound salesplay which has expired.
- A need to act on results to build a new sequence or cadence which can’t wait.
- Limited number of prospects who qualify for a sequence or cadence, and you’ve contacted all of them.
Or, you might be working with a hypothesis that is broader than a race between two templates. As an example, you might be looking at the difference it would make to add or subtract a call step in a sequence or cadence.
This would require cloning the sequence, adding or subtracting that call step, and watching the results. But because SEPs don’t currently have the functionality to monitor these experiments, you’re on your own to evaluate outcomes.
Thankfully, because we assume you’re not a math professor (and neither are we), there are free calculators out there which will crunch these numbers for you. Here is a good one, which you can include in your sales messaging playbook or other documentation on A/B testing.
You enter your opens, clicks, or replies for A, the same for B, and then the sample size (number of sends). This calculator will–like an SEP or marketing automation platform–crunch the numbers for you.
It will tell you, for instance, if there have been enough sends, or if you need a larger sample size. If you have a large enough sample size, it will tell you how confident you can be (look for 95% or higher statistical significance) and the “uplift,” or the difference your change made. While there isn’t a specific target here, the higher the uplift percentage, the more impactful your change was.
Here’s what to do if you just can’t get enough sends
If your team is really in a pickle, and true statistical significance isn’t possible, then there are some basic guidelines for declaring a winner. We have to add the caveat, however, that these results should not be given the same authority as statistically significant results.
These results won’t be a “law” you should document in your sales messaging playbook; they’re more like a confident theory which should be tested again as soon as possible but may inform message creation in the meantime.
Here are the “in a pinch” criteria:
- At least 100 sends in each variant AND
- A noticeable difference between your A and B tests.
What counts as “noticeable” will be different in each case, but you will have a built-in definition for this, if your hypothesis is specific enough. For instance, if you determine that “good” looks like an increase in open rates by 2%, then wait until that difference has been met or exceeded. If you haven’t hit that mark, then your hypothesis is likely either not correct or needs to be tested again with a new experiment.
Pro Tip: Don’t abandon A/B testing too soon
We have met more than a few sales teams who have tried A/B testing, but they didn’t see the value they were expecting. The lessons learned weren’t earth-shattering, so they stopped.
If this happens, you might be doing it wrong. With a strong hypothesis, properly-framed experiment, and well-isolated variables, it’s reasonable to expect that your team is going to walk away with an actionable outcome.
If not, then you’re probably not asking the right questions or you’re not sticking with your experiments long enough to see reliable results.
Here’s what you should do next
Sales leaders have a strong stake in how well their sales messaging teams A/B test, because they’re often in decision-making chairs, trying to make choices about how to enable their sales teams with the best possible messages.
Even if your next step is sending this piece to your sales messaging creators, you should stay involved in the process to ensure that your team takes the needed steps to create and execute on an A/B testing framework.
Without one, you can’t be confident that your sales reps are putting their best feet forward with your prospects and accounts.
Here are your next steps to establishing that confidence:
- Take a look at any active A/B tests in your sales or marketing automation program.
- Look at the results and research whether your team has acted on those learnings.
- Sit down with your sales messaging teams to discuss what hypotheses to prioritize for future testing.
- Invite a “Greaser” to the table. We can help you choose and build experiments to answer your most burning questions.
- Download our sales messaging playbook template, which will help your team document and share your findings.